Updating Purpose Limitation for AI
Joint research project by the philosopher Rainer Mühlhoff and the legal scholar Hannah Ruschemeier.
The project reacts to the risk of secondary use of trained AI models, which is currently one of the most severe regulatory gaps with regard to AI. Purpose Limitation for Models limits the use of trained AI models to the purpose for which it was originally trained and for which the training data was collected.
See also the Predicive Privacy project.
The risk of secondary use of trained models
Imagine medical researchers build an AI model that detects depression from speech data. Let’s say the model is trained from the data of volunteer psychiatric patients. This could be a beneficial project to improve medical diagnosis, and that’s why many consent to the use of their data. But what if the trained model falls into the hands of the insurance industry or of a company that builds AI systems to evaluate job interviews? In these cases, the model would facilitate implicit discrimination of an already vulnerable group.
There are currently no effective legal limitations to reusing trained models for other purposes (this includes the forthcoming AI Act). Secondary use of trained models poses an immense societal risk and a blind spot of ethical and legal debate.
The risk of misuse of trained models
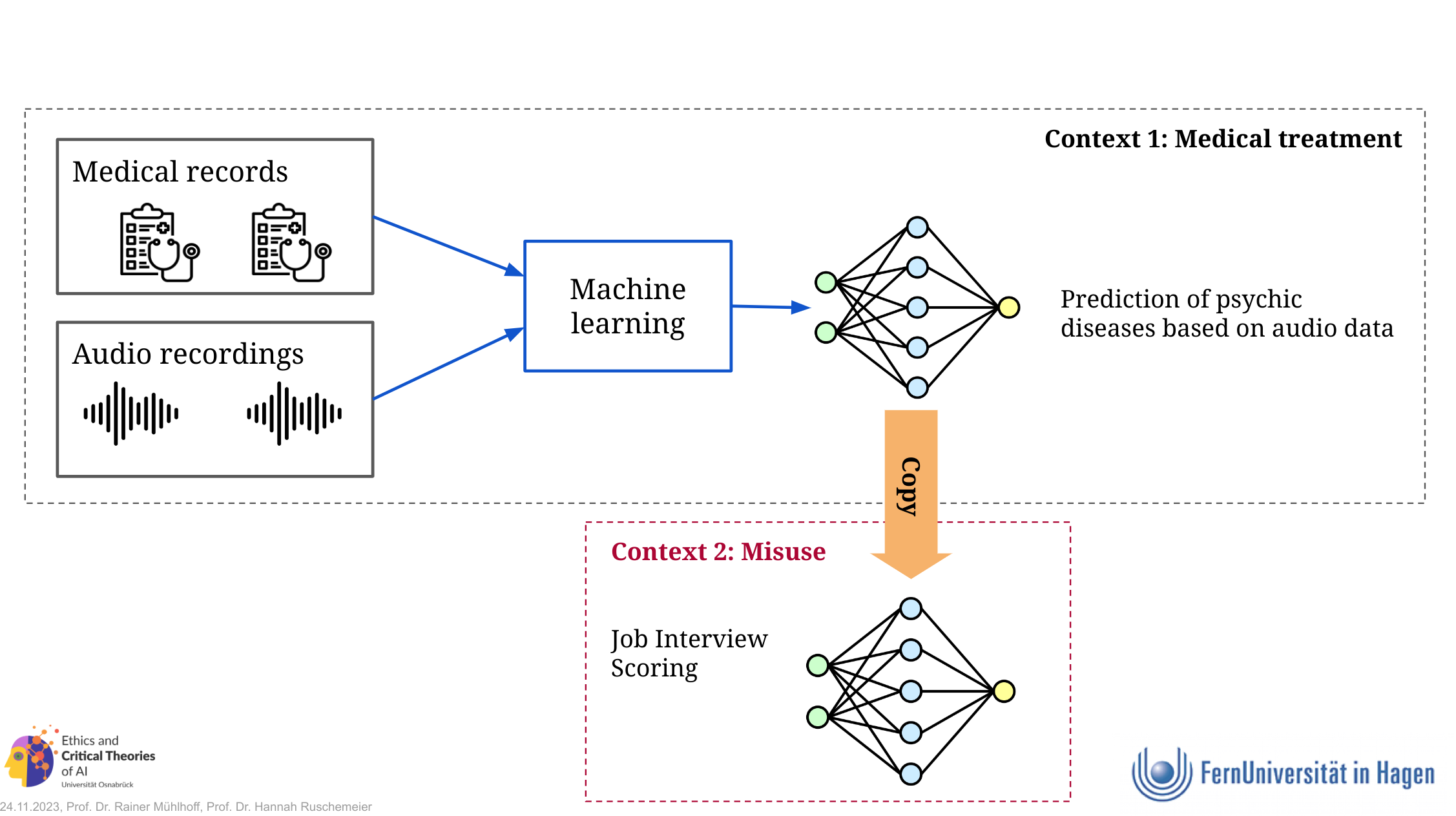
What is Purpose Limitation for AI?
In our interdisciplinary project combining critical AI ethics and legal studies, we develop the concept of Purpose Limitation for Models as an ethical and legal framework to govern the purposes for which trained models (and training dataset) may be used and reused.
The concept comes in two variations that state:
- A machine learning model shall only be trained, used and transferred for the purposes for which the training data was collected.
- An actor shall only train a machine learning model from a data set if the purposes for which the data set was originally collected is compatible with the purpose for which the model is trained.
Purpose limitation is originally a concept from data protection regulation (Art 5(1)(b) GDPR), but it does not automatically apply to the training and/or further processing of AI models. This is because AI models can be trained from anonymised training data and in many relevant cases, the model data itself might be anonymous data. The GDPR does not apply to the processing of anonymous data.
Two variations of PL for AI
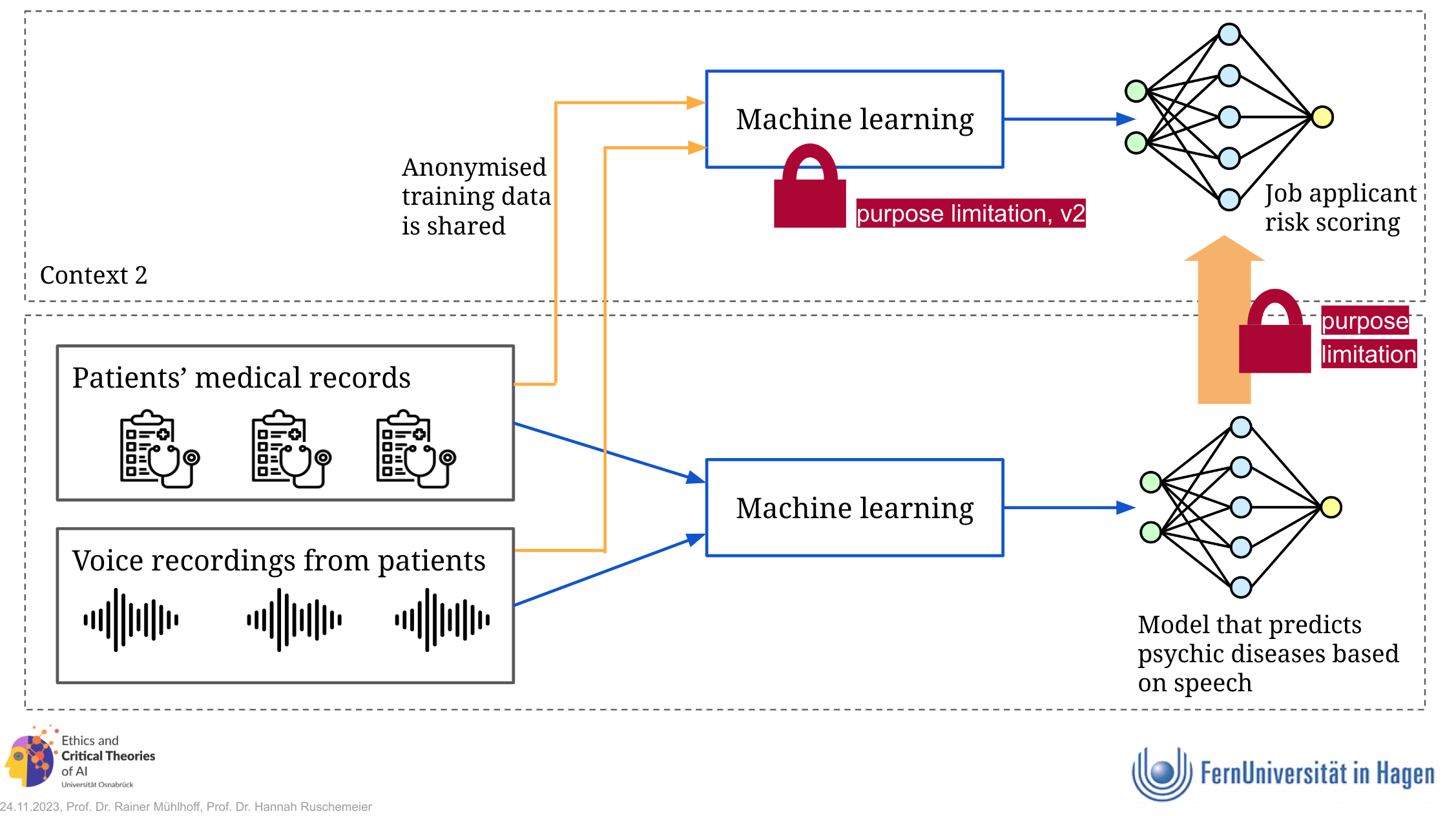
Why is Purpose Limitation for AI important?
We argue that possession of trained models is at the core of an increasing asymmetry of informational power between AI companies and society. Limiting this power asymmetry must be the goal of regulation, and Purpose Limitation for Models would be a key step in that direction. The production of predictive and generative AI models signifies the most recent form of informational power asymmetry between data processing organisations (mostly large companies) and societies. Without public control of the purposes for which existing AI models can be reused in other contexts, this power asymmetry poses significant individual and societal risks in the form of discrimination, unfair treatment, and exploitation of vulnerabilities (e.g., risks of medical conditions being implicitly estimated in job applicant screening). Our proposed purpose limitation for AI models aims to establish accountability, effective oversight, and prevent collective harms related to the regulatory gap.
Does the AI Act prevent the risk of secondary use of trained models?
The AI Act does not regulate AI models in the training phase, but only applies when they are placed on the market. Following this, the AI Act’s risk categorisation is based on a dichotomy of product safety risks and fundamental rights risks, where the secondary use of models is not considered a risk factor. Consequently, the secondary use of models plays no role in the AI Act’s risk assessment of an AI system. The AI Act’s requirements for the creation of a database of high-risk systems can be used as a starting point for a governance structure for our proposal of a Purpose Limitation for AI.
Research articles
on Purpose Limitation for Models
-
Mühlhoff, Rainer, und Hannah Ruschemeier. 2024. „Regulating AI via Purpose Limitation for Models“. AI Law and Regulation. https://dx.doi.org/10.21552/aire/2024/1/5.
Full text Publisher's website bibtex
×@article{Mü-Ru2023:PLM, title = {Regulating {{AI}} via {{Purpose Limitation}} for {{Models}}}, author = {Mühlhoff, Rainer and Ruschemeier, Hannah}, journal = {AI Law and Regulation}, url = {https://dx.doi.org/10.21552/aire/2024/1/5}, year = {2024}, langid = {english}, web_group = {aktuell}, web_thumbnail = {/assets/images/publications/Mü-Ru2023:PLM.jpg}, web_fulltext = {/media/publications/mühlhoff_ruschemeier_2024_regulating_ai_with_purpose_limitation_for_models.pdf}, web_publisher = {https://aire.lexxion.eu/article/AIRE/2024/1/5} }
-
Mühlhoff, Rainer, und Hannah Ruschemeier. 2024. „Updating Purpose Limitation for AI: A Normative Approach from Law and Philosophy“. SSRN Preprint, Januar. https://papers.ssrn.com/abstract=4711621.×
@article{Mü-Ru2024:UPL, type = {SSRN Preprint}, title = {Updating {{Purpose Limitation}} for {{AI}}: {{A}} Normative Approach from Law and Philosophy}, shorttitle = {Updating {{Purpose Limitation}} for {{AI}}}, author = {Mühlhoff, Rainer and Ruschemeier, Hannah}, date = {2024-01-22}, url = {https://papers.ssrn.com/abstract=4711621}, langid = {english}, pubstate = {preprint}, keywords = {AI Act,AI Governance,AI regulation,collective privacy,data ethics,data protection,Ethics,EU regulation,GDPR,general purpose AI systems,LLMSs,Open Source,power asymmetries,secondary data use}, web_thumbnail = {/assets/images/publications/Mü-Ru2024:UPL.jpg}, web_fulltext = {/media/publications/mühlhoff_ruschemeier_2024_updating_purpose_limitation_for_ai.pdf}, web_preprint = {https://ssrn.com/abstract=4711621}, web_group = {preprint} }
On the risk of secondary use of trained models
-
Ruschemeier, Hannah. 2024. „Prediction Power as a Challenge for the Rule of Law“. SSRN Preprint. doi:10.2139/ssrn.4888087.×
@article{Ru2024:PredictionPower, type = {SSRN Preprint}, title = {Prediction Power as a Challenge for the Rule of Law}, author = {Ruschemeier, Hannah}, year = {2024}, doi = {10.2139/ssrn.4888087}, url = {https://doi.org/10.2139/ssrn.4888087}, langid = {english}, keywords = {AI,Data,Power,Predictions,Public Actors,Rule of Law}, pubstate = {preprint}, web_fulltext = {https://papers.ssrn.com/abstract=4814999}, web_group = {third-party}, web_thumbnail = {/assets/images/publications/Ru2024:PredictionPower.jpg} }
-
Mühlhoff, Rainer. 2024. „Das Risiko der Sekundärnutzung trainierter Modelle als zentrales Problem von Datenschutz und KI-Regulierung im Medizinbereich“. In KI und Robotik in der Medizin – interdisziplinäre Fragen, herausgegeben von Hannah Ruschemeier und Björn Steinrötter. Nomos. doi:10.5771/9783748939726-27.×
@incollection{Mü2023RisikoSekundärnutzung, title = {Das Risiko der Sekundärnutzung trainierter Modelle als zentrales Problem von Datenschutz und KI-Regulierung im Medizinbereich}, booktitle = {KI und Robotik in der Medizin – interdisziplinäre Fragen}, author = {Mühlhoff, Rainer}, editor = {Ruschemeier, Hannah and Steinrötter, Björn}, year = {2024}, doi = {10.5771/9783748939726-27}, publisher = {Nomos}, isbn = {9783748939726}, web_group = {aktuell}, web_thumbnail = {/assets/images/publications/Mü2023RisikoSekundärnutzung.jpg}, web_fulltext = {/media/publications/mühlhoff_2024_risiko_der_sekundärnutzung.pdf} }
-
Mühlhoff, Rainer, und Theresa Willem. 2023. „Social Media Advertising for Clinical Studies: Ethical and Data Protection Implications of Online Targeting“. Big Data & Society, 1–15. doi:10.1177/20539517231156127.×
@article{Mü-Willem2022, author = {Mühlhoff, Rainer and Willem, Theresa}, title = {Social Media Advertising for Clinical Studies: Ethical and Data Protection Implications of Online Targeting}, journal = {Big Data & Society}, page = {1–15}, year = {2023}, doi = {10.1177/20539517231156127}, web_group = {papers}, web_thumbnail = {/assets/images/publications/Mü-Willem2022.jpg}, web_fulltext = {/media/publications/mühlhoff_willem_2023_social_media_advertising_for_clinical_studies.pdf} }
-
Mühlhoff, Rainer. 2023. Die Macht der Daten. Warum Künstliche Intelligenz eine Frage der Ethik ist. V&R unipress, Universitätsverlag Osnabrück. doi:10.14220/9783737015523.
Full text Publisher's website bibtex
×@book{Mü2023:MD, title = {Die Macht der Daten. Warum Künstliche Intelligenz eine Frage der Ethik ist}, author = {Mühlhoff, Rainer}, doi = {10.14220/9783737015523}, isbn = {978-3-8471-1552-6,978-3-7370-1552-3}, publisher = {V&R unipress, Universitätsverlag Osnabrück}, url = {https://www.vr-elibrary.de/doi/book/10.14220/9783737015523}, year = {2023}, web_group = {books}, web_thumbnail = {/assets/images/publications/Mü2023:MD.jpg}, web_fulltext = {/media/publications/mühlhoff_2023_die_macht_der_daten.pdf}, web_publisher = {https://www.vandenhoeck-ruprecht-verlage.com/themen-entdecken/schule-und-unterricht/ethik-werte-und-normen/58171/die-macht-der-daten} }
On the limitations of existing data protection regulation
-
Mühlhoff, Rainer, und Hannah Ruschemeier. 2024. „Predictive Analytics and the GDPR: Collective Dimensions of Data Protection“. Law, Innovation and Technology. doi:10.1080/17579961.2024.2313794.×
@article{Mü-Ru2024:LIT, title = {Predictive {{Analytics}} and the {{GDPR}}: {{Collective Dimensions}} of {{Data Protection}}}, author = {Mühlhoff, Rainer and Ruschemeier, Hannah}, date = {2024}, journaltitle = {Law, Innovation and Technology}, doi = {10.1080/17579961.2024.2313794}, annotation = {alter key: Mü-Ru2023:EN}, web_group = {papers}, web_thumbnail = {/assets/images/publications/Mü-Ru2024:LIT.jpg}, web_fulltext = {/media/publications/mühlhoff_ruschemeier_2024_predictive_analytics_and_the_gdpr.pdf} }
-
Ruschemeier, Hannah. 2024. „Generative AI and Data Protection“. SSRN Preprint. https://papers.ssrn.com/abstract=4814999.×
@article{Ru2024:GenAI, type = {SSRN Preprint}, title = {Generative AI and Data Protection}, author = {Ruschemeier, Hannah}, year = {2024}, url = {https://papers.ssrn.com/abstract=4814999}, langid = {english}, pubstate = {preprint}, keywords = {AI,AI Act,Data Protection,EU Data Protection,GDPR,Generative AI,LLMs,privacy,privacy rights,regulation,right to data protection}, web_thumbnail = {/assets/images/publications/Ru2024:GenAI.jpg}, web_fulltext = {https://papers.ssrn.com/abstract=4814999}, web_group = {third-party} }
-
Mühlhoff, Rainer, und Hannah Ruschemeier. 2022. „Predictive Analytics und DSGVO: Ethische und rechtliche Implikationen“. In Telemedicus – Recht der Informationsgesellschaft, Tagungsband zur Sommerkonferenz 2022, herausgegeben von Hans-Christian Gräfe und Telemedicus e.V., 38–67. Deutscher Fachverlag.×
@incollection{Mü-Ru2022, title = {Predictive Analytics und DSGVO: Ethische und rechtliche Implikationen}, booktitle = {Telemedicus – Recht der Informationsgesellschaft, Tagungsband zur Sommerkonferenz 2022}, author = {Mühlhoff, Rainer and Ruschemeier, Hannah}, editor = {Gräfe, Hans-Christian and {Telemedicus e.V.}}, date = {2022}, pages = {38--67}, publisher = {Deutscher Fachverlag}, location = {Frankfurt am Main}, isbn = {978-3-8005-1857-9}, web_group = {papers}, web_thumbnail = {/assets/images/publications/Mü-Ru2022.jpg}, web_fulltext = {/media/publications/telemedicus-2022-tagungsband-isbn-978-3-8005-1857-9.pdf} }